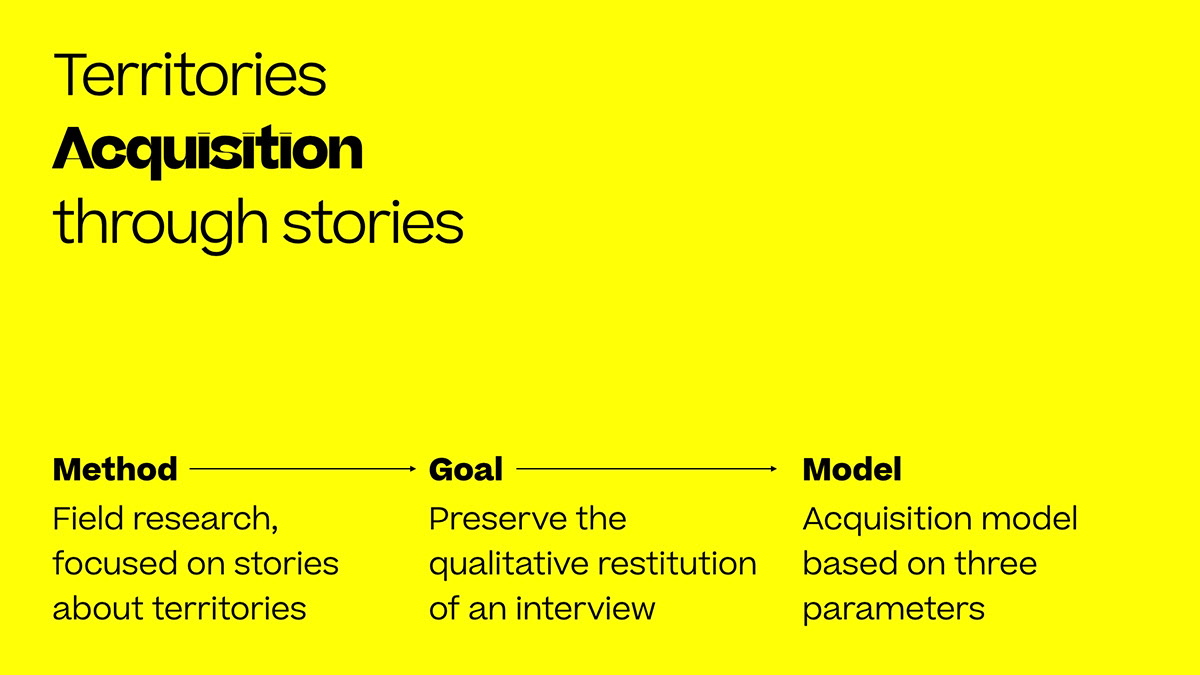
QUALITATIVE VOICE RECOGNITION (pag. 8-9)
EXCERPT from Cumulus Conference Proceedings Roma 2020-2021 | Track: Design Culture (of) LANGUAGES – Beyondstories
Nowadays, automatic speech-to-text recognition and manual unwinding are the main ways to get a transcription from a recorded audio. Speech-to-text recognition is fast and increasingly precise, but it has limits in the restitution of a narrative content, not providing indications in emotional terms. The unwinding allows a more qualitative analysis of a text/audio, but it is a slow and expensive process.
In order to provide technological support to the physical action of the story-finder, we designed a model divided into steps, with the aim of obtaining qualitative and narrative data from voice recognition.
Phase 1
During the interview, the story-finder puts keyframes of fixed duration (2-3 minutes). The keyframes are parts of the entire story that become autonomous micro-stories,
with narrative value and - if possible - a self-concluding sense. Each micro-story is an agglomeration of the words that composed it.
with narrative value and - if possible - a self-concluding sense. Each micro-story is an agglomeration of the words that composed it.
Phase 2
Each micro-story is analyzed by voice recognition, enhanced by the addition of three parameters: Time, Narrator and Emotion.
• Time
Compared to the time x of the interview, what time y does the narration refer to? The “present” axis is not at the center because - based on field research results - it emerged that memories are prevalent within an interview.
Compared to the time x of the interview, what time y does the narration refer to? The “present” axis is not at the center because - based on field research results - it emerged that memories are prevalent within an interview.
• Narrator
Does the person talk about himself (selfdiegetic), his context (homodiegetic) or with an observer's eye (heterodiegetic)?
Does the person talk about himself (selfdiegetic), his context (homodiegetic) or with an observer's eye (heterodiegetic)?
• Emotion
Towards which axes does the emotional substrate of the narrative tend? Emotions are shown as configurational situations, resonances of human behavior, not polarized and with a nuanced nature. The poles from which the infinite emotional nuances spring up concern the perceived sensation (vital- nefarious) and the temporal connotation (present-expected) of emotions. Time and narrator parameters come from Genette's studies on narratology ("Gerard Genette", 2019). The emotion parameter is a union between Lisa Feldman Barrett's neuroscientific studies (Della Rocca, 2019) and Umberto Galimberti's philosophical analysis (Galimberti, 2019).
Towards which axes does the emotional substrate of the narrative tend? Emotions are shown as configurational situations, resonances of human behavior, not polarized and with a nuanced nature. The poles from which the infinite emotional nuances spring up concern the perceived sensation (vital- nefarious) and the temporal connotation (present-expected) of emotions. Time and narrator parameters come from Genette's studies on narratology ("Gerard Genette", 2019). The emotion parameter is a union between Lisa Feldman Barrett's neuroscientific studies (Della Rocca, 2019) and Umberto Galimberti's philosophical analysis (Galimberti, 2019).
Phase 3
Adding the three parameters, every word of each micro-story is categorized and expanded semantically. Each word will have a wide range of connections with other words and at the same time a more specific connotation depending on the parameter. All the micro-stories, divided by topic and categorized by parameters, create the Beyondstories database.
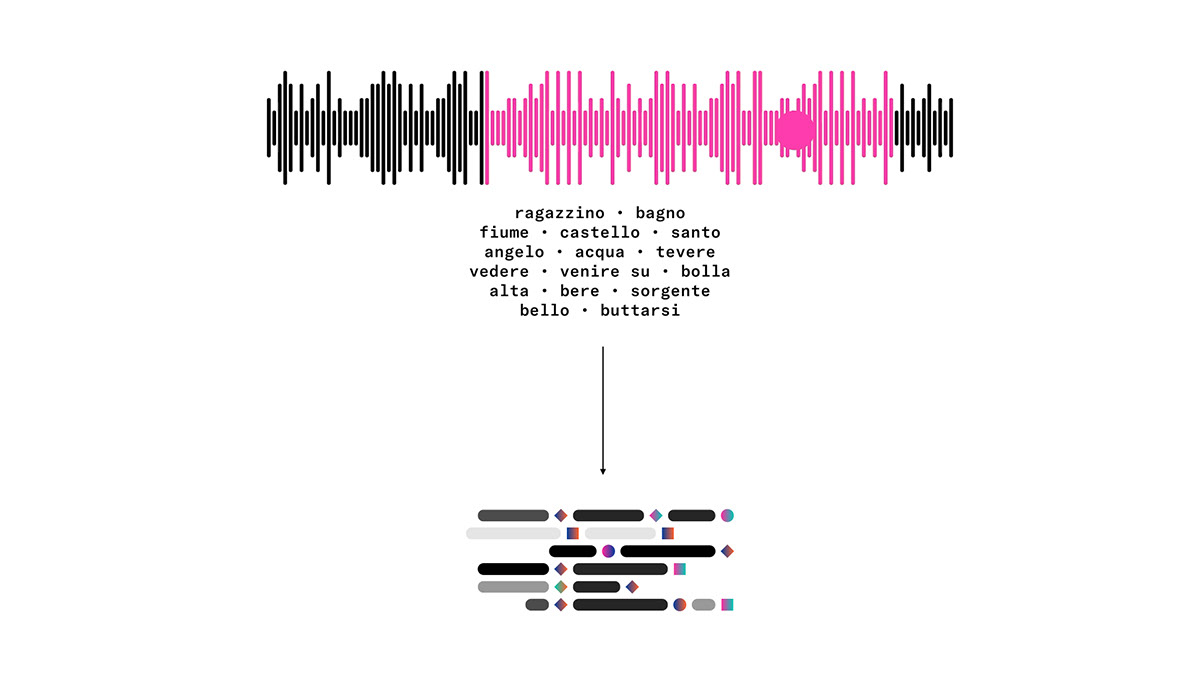
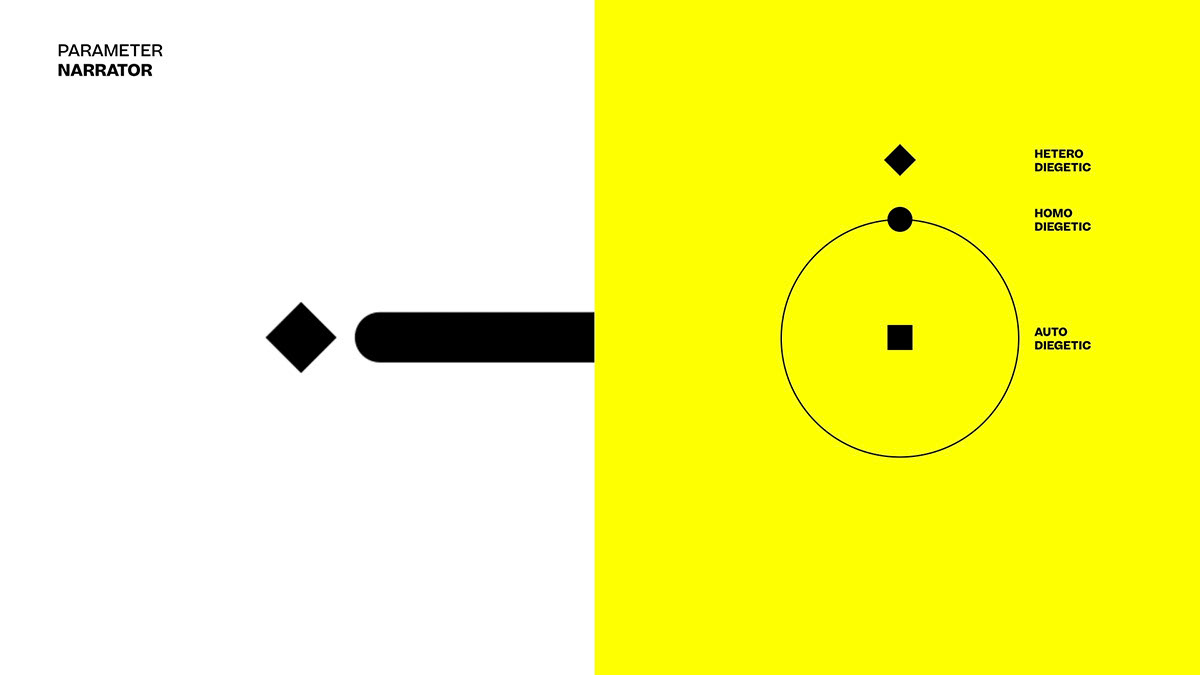
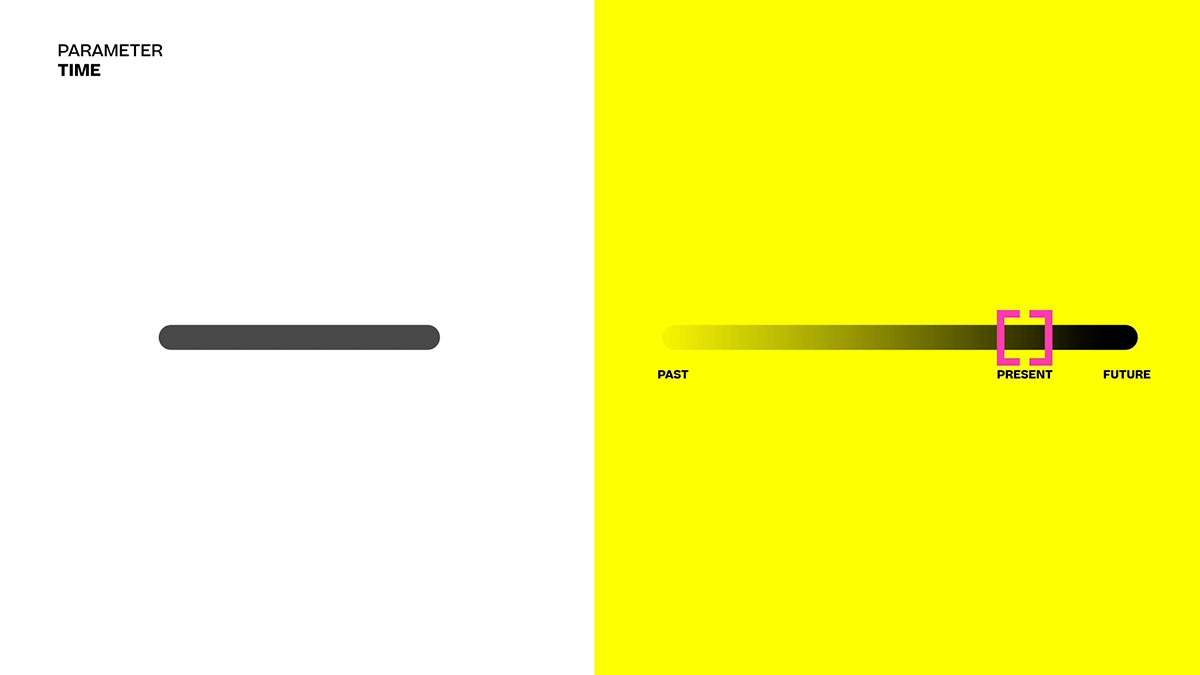
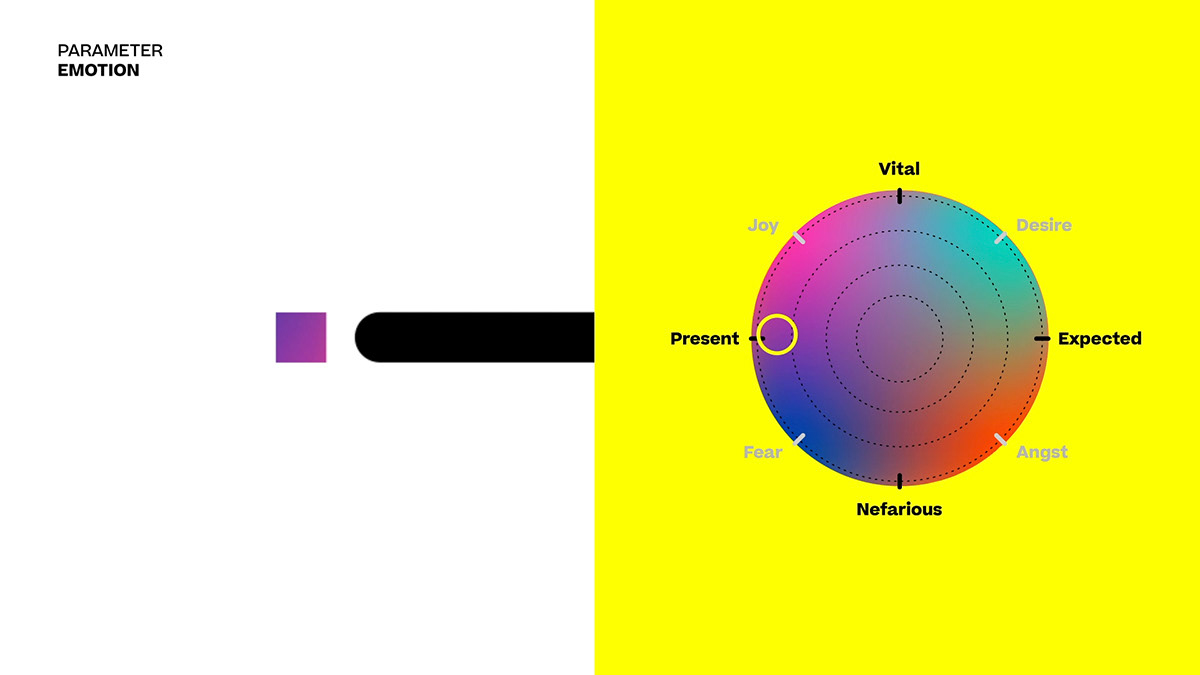
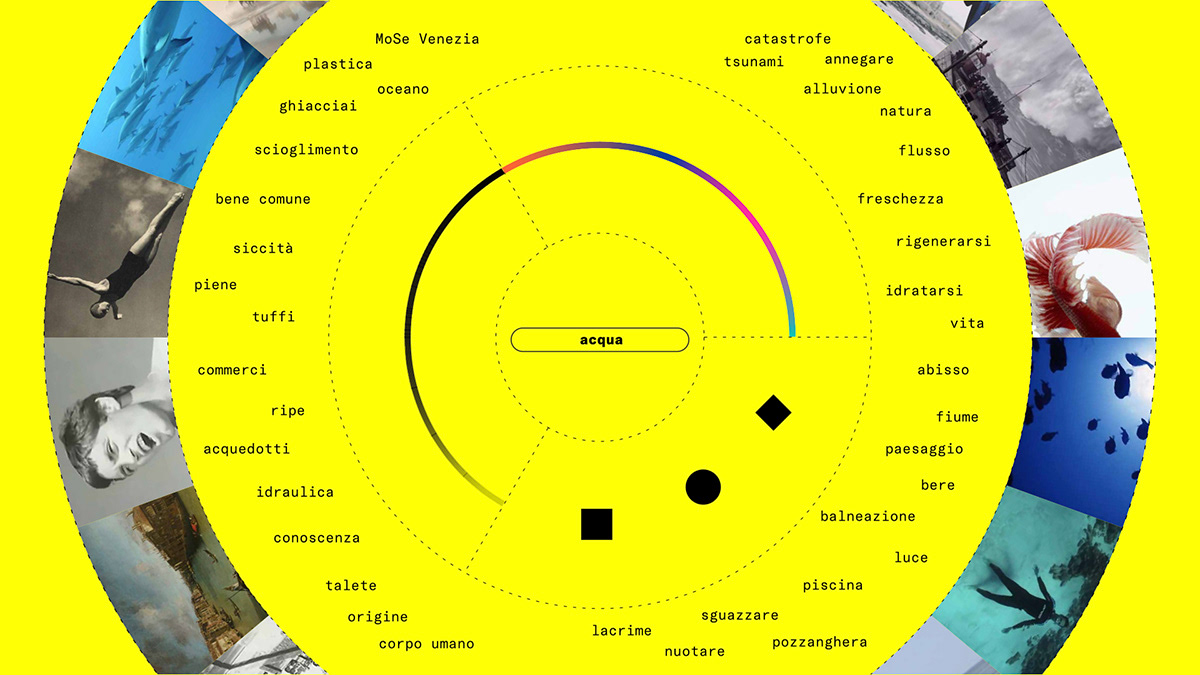
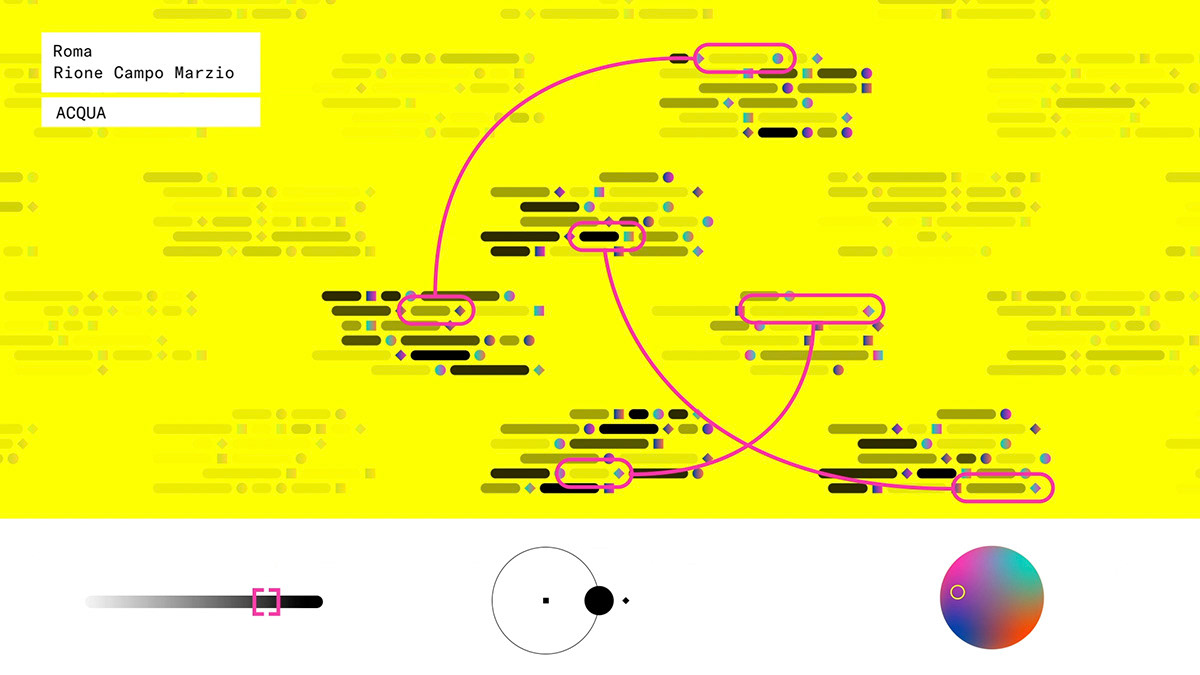